Processes that occur at a finer scale than those captured by climate models, such as large-scale ocean circulation or “eddies,” are represented by parameterizations—a set of descriptions that allow the models to incorporate those processes without a large computational cost. However, parameterizations often reduce the accuracy of climate simulations. Research funded in part by CPO’s Climate Variability & Predictability (CVP) program shows that machine learning techniques can improve climate model simulations with efficient parameterizations that more accurately represent ocean currents in models. The study, published in Geophysical Research Letters, tested two machine learning methods: relevance vector machines (RVMs) and convolutional neural networks (CNNs). The authors found that while CNN showed higher skill in reproducing the model simulation, the mechanisms guiding RVM were more interpretable.
Machine Learning Offers New Tools to Improve Ocean Climate Models
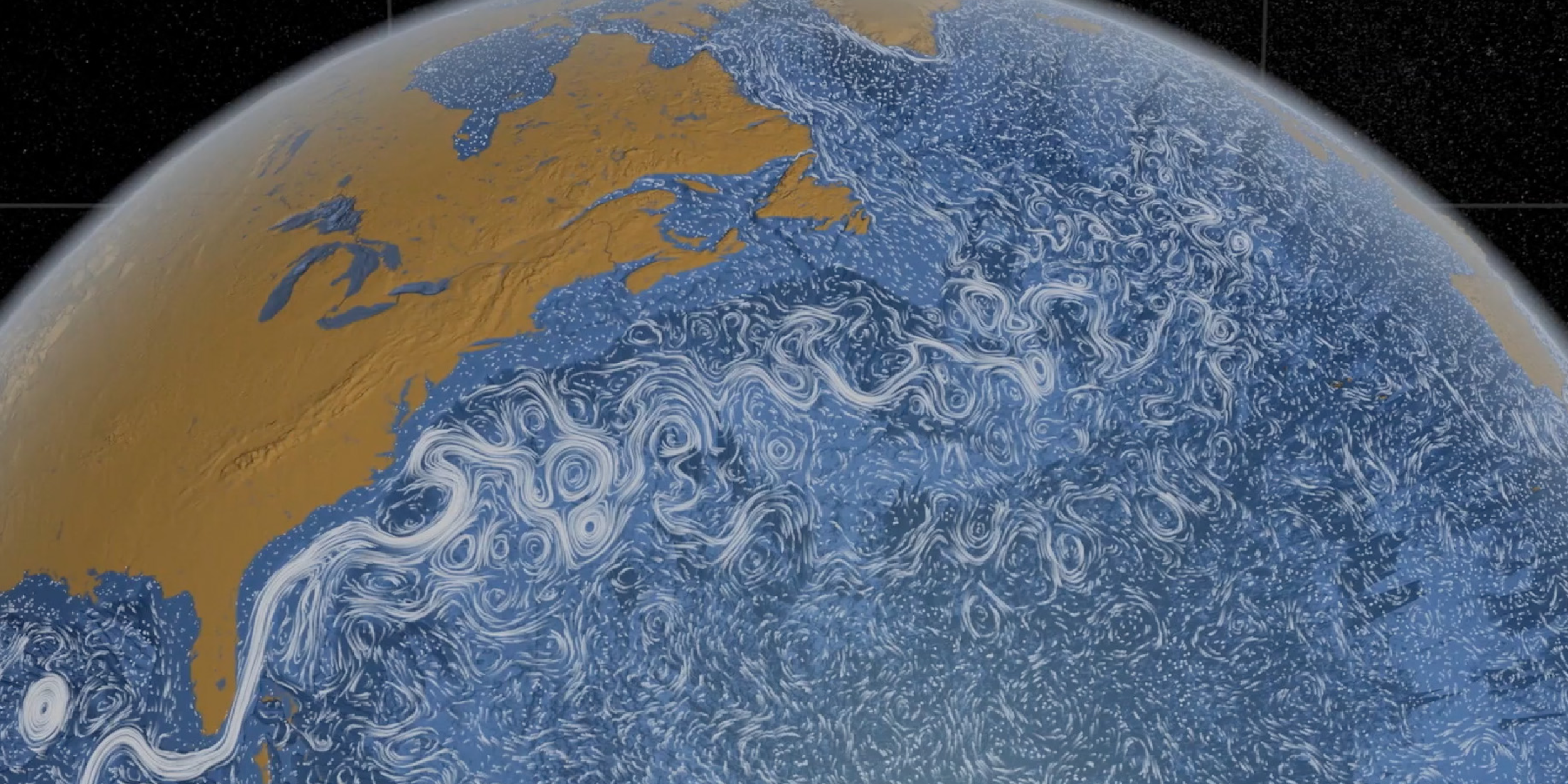
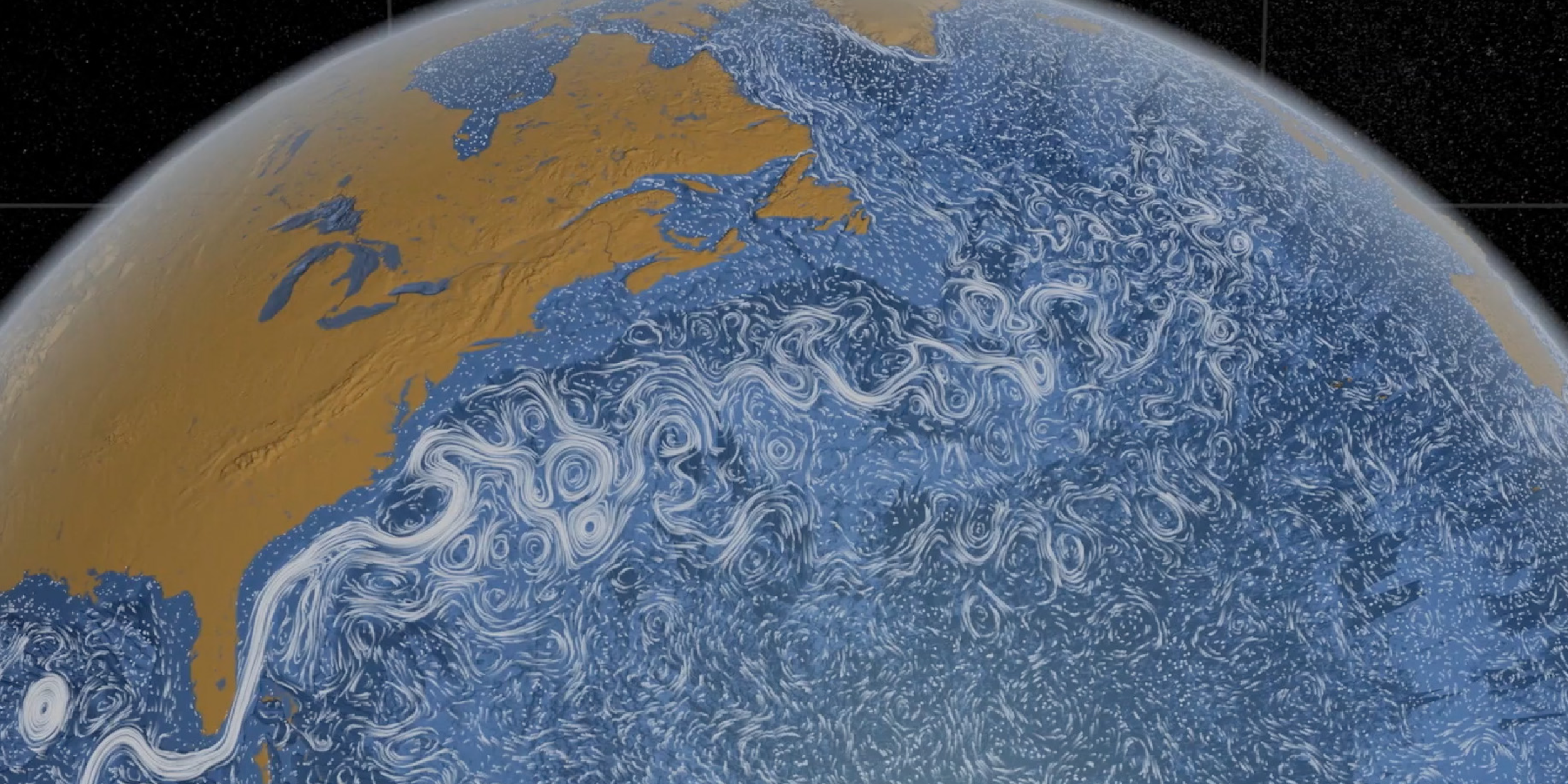