As the Arctic Ocean’s sea ice cover continues to decrease, scientists have been working to better understand what drives sea ice loss to improve predictions of seasonal changes in sea ice. A new study supported by CPO’s Climate Variability and Predictability (CVP) program, led by CIRES and the University of Colorado Boulder, uses maps built with machine learning to identify large-scale atmospheric patterns that are linked with the start of seasonal sea ice melt in the Arctic. Knowing what triggers these pressure patterns offers a potential source of seasonal predictability for early melt onset. The study is published in Climate Dynamics. Early sea ice melt is often triggered by increased radiation associated with increased levels of moisture in the atmosphere. Scientists have already confirmed that the changes in moisture are connected to atmospheric transport from remote areas, rather than transport from the Arctic surface itself. Using self-organizing maps, a type of machine learning, the researchers mapped melt onset dates on to daily sea level pressure from 1979-2018 during melt season (April-July) over the Arctic. Sean Horvath, Julienne Stroeve, Balaji Rajagopalan, and Alexandra Jahn found that circulation patterns drive much of the moisture transport to the Arctic. The patterns are variations on a broad low pressure region stretching over Siberia and a high pressure region over North America and Greenland, which funnel moisture from lower latitudes and through the Bering and Chukchi Seas. According to the study, the early or late start of sea ice melt depends on whether strong or weak circulation patterns emerge, respectively. Read the article »
Machine Learning Reveals Links between Atmospheric Pressure Patterns and Arctic Sea Ice Melt
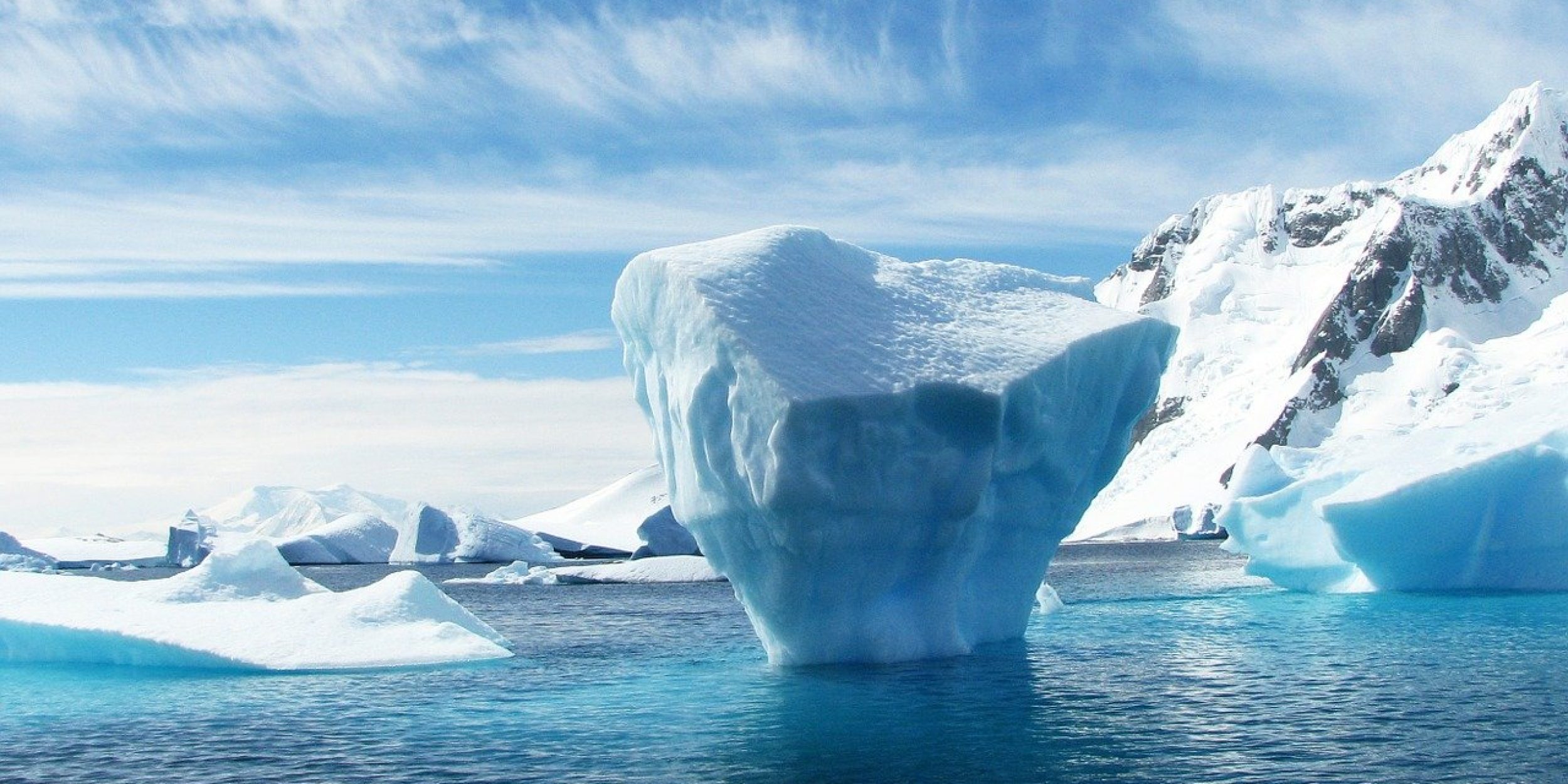
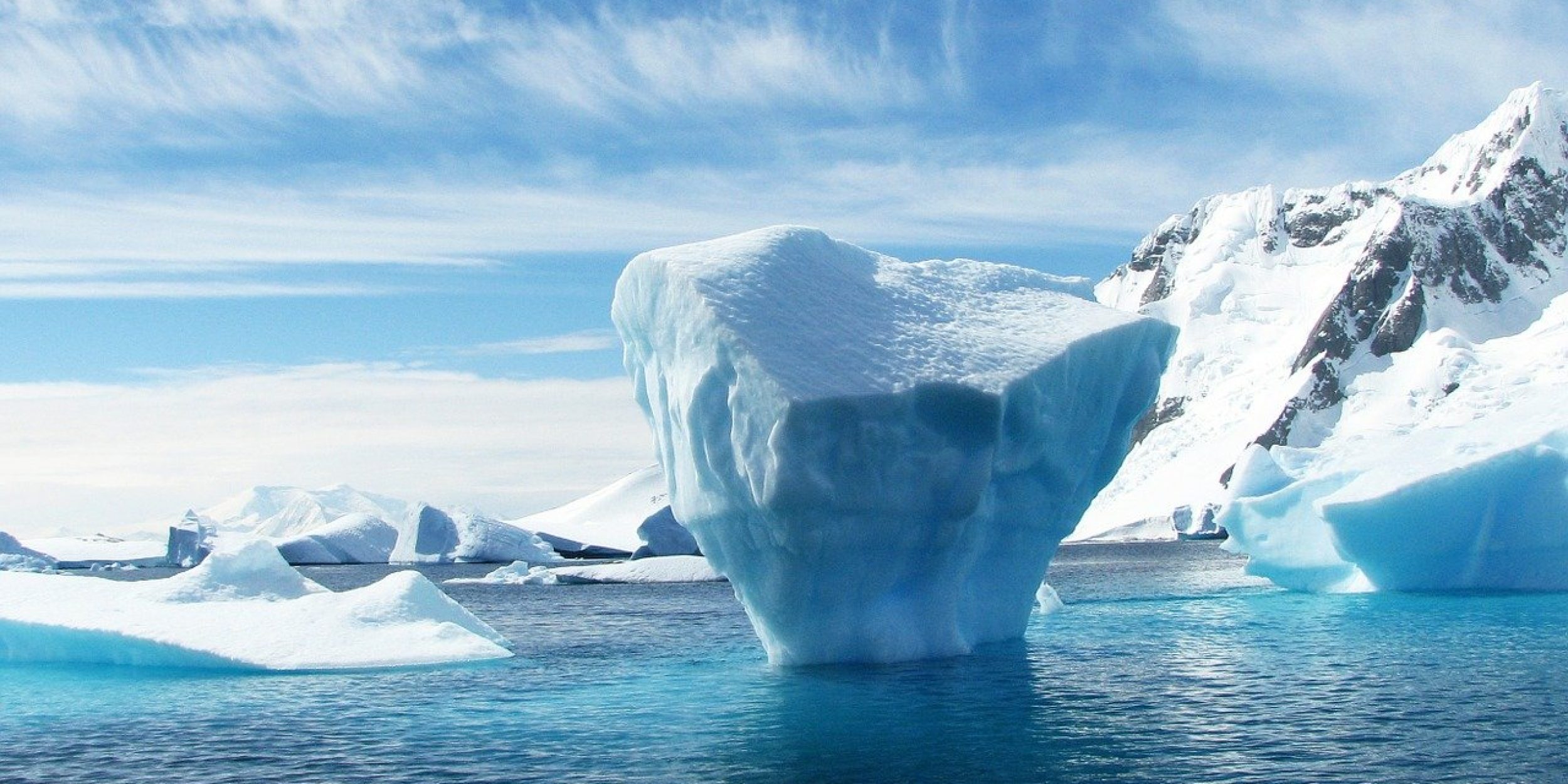